The emergence of artificial intelligence (AI) and machine learning (ML) technologies has drastically transformed numerous industries, and healthcare is no exception. One of the most exciting and potentially life-saving applications of these technologies is in drug discovery and development. LLM knowledge graph drug purpose has become a crucial area of research and application, enabling the discovery of new drugs, the identification of existing treatments for novel diseases, and the optimization of drug repurposing efforts. This article will explore the concept of LLM knowledge graph drug purpose, its significance, and how it is revolutionizing the pharmaceutical industry.
Table of Contents
What is an LLM Knowledge Graph?
A knowledge graph is a data structure that uses nodes, edges, and labels to represent entities and their relationships in a network. The idea is to model knowledge in a way that mimics human understanding, making it easier to reason about complex relationships between concepts. A LLM knowledge graph drug purpose is a specialized knowledge graph that integrates information related to pharmaceuticals, including drug properties, molecular structures, clinical outcomes, side effects, and more.
Large Language Models (LLMs) are AI models designed to process and generate natural language. When combined with knowledge graphs, LLMs can analyze vast amounts of scientific literature, drug data, and clinical trial results, providing deep insights into drug mechanisms, potential interactions, and therapeutic purposes. The LLM knowledge graph drug purpose helps researchers understand how drugs can be utilized to treat various conditions and how existing drugs might be repurposed for new therapeutic purposes.
The Role of LLM Knowledge Graph Drug Purpose in Drug Discovery
The drug discovery process is long, expensive, and fraught with uncertainty. Identifying viable drug candidates involves numerous stages, including target identification, compound screening, preclinical testing, and clinical trials. Each of these steps can take years, and the probability of failure is high. By leveraging an LLM knowledge graph drug purpose, researchers can significantly streamline this process.
- Drug Target Identification: One of the first steps in drug discovery is identifying a biological target, such as a protein or gene, that a drug can interact with to treat a disease. An LLM knowledge graph drug purpose can help researchers identify new targets by mining scientific literature, genetic databases, and experimental data. The graph can highlight previously unnoticed links between diseases, genes, and molecular pathways, opening up new avenues for therapeutic exploration.
- Drug Repurposing: Repositioning existing drugs for new indications is a cost-effective and time-saving strategy. LLM knowledge graph drug purpose aids in drug repurposing by identifying drugs that might work against diseases beyond their original intended purpose. By analyzing data from clinical trials, genetic studies, and pharmacological information, the graph can predict new uses for existing drugs. For example, a drug initially developed for cancer might also show promise in treating neurodegenerative diseases.
- Predicting Drug Efficacy and Toxicity: The challenge of predicting how well a drug will work and what side effects it might have is one of the biggest hurdles in drug development. An LLM knowledge graph drug purpose can assist in this prediction by integrating data from various sources, such as animal models, human trials, and molecular studies. By analyzing this data, researchers can gain a better understanding of a drug’s potential effectiveness and safety profile before proceeding with costly clinical trials.
How Does an LLM Knowledge Graph Work in Drug Research?
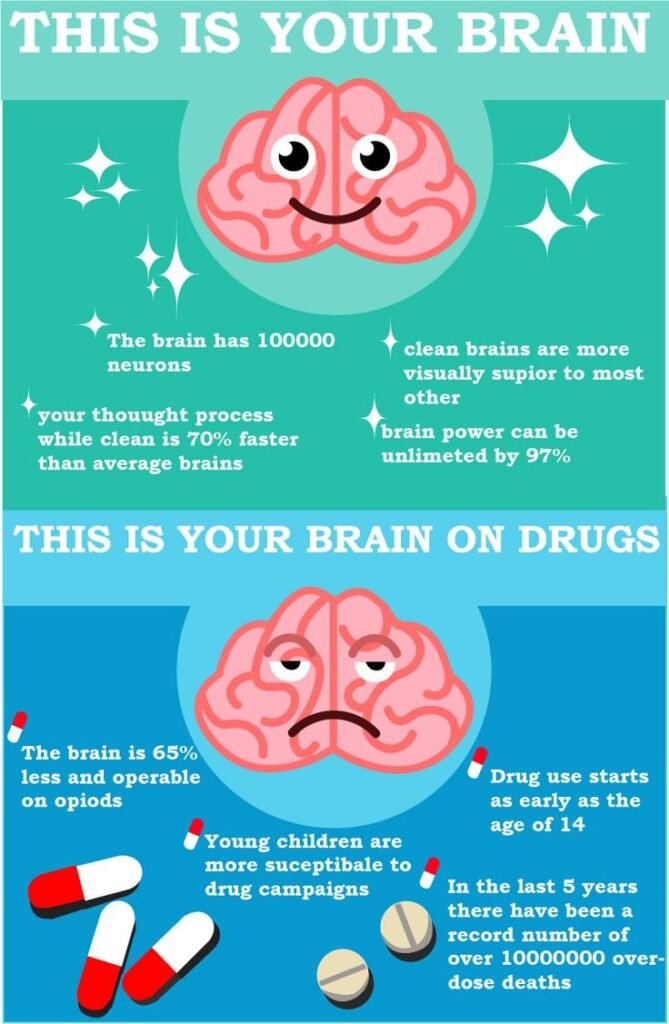
To understand the functionality of an LLM knowledge graph drug purpose, it’s essential to break down how it operates. An LLM-powered knowledge graph uses vast amounts of scientific and medical data, which is then processed by large language models to make sense of the information and create connections between entities.
- Data Integration: The first step involves aggregating data from diverse sources, including clinical trial reports, genetic databases, chemical compound repositories, and research papers. The LLM knowledge graph drug purpose pulls in information from multiple disciplines, including genomics, pharmacology, and toxicology.
- Entity Recognition and Relationship Extraction: Once the data is gathered, the LLM model identifies entities such as drugs, diseases, genes, proteins, and chemical compounds. These entities are then linked to each other in a knowledge graph, showing the relationships between them. For example, the graph might reveal that a particular drug interacts with a specific protein involved in cancer development, suggesting a potential new therapeutic use for the drug.
- Pattern Recognition and Insight Generation: The power of LLMs lies in their ability to identify complex patterns within data. The LLM knowledge graph drug purpose analyzes the graph’s structure and relationships, offering insights that can guide drug discovery and development efforts. Researchers can use these insights to prioritize drug candidates, predict their effects, and identify promising therapeutic areas.
- Natural Language Processing: One of the key advantages of using LLMs is their ability to process and generate human-like language. With natural language processing (NLP) capabilities, an LLM knowledge graph drug purpose can translate complex data into actionable insights in a manner that is understandable to scientists and researchers. It can generate reports, summaries, and recommendations based on the information within the graph, aiding decision-making throughout the drug development process.
Applications of LLM Knowledge Graph Drug Purpose in Modern Healthcare
The integration of LLM-powered knowledge graphs in drug discovery and development offers a range of benefits that go beyond just speeding up the process. These systems have the potential to revolutionize healthcare by providing better-targeted therapies, reducing costs, and improving patient outcomes.
- Personalized Medicine: One of the ultimate goals of modern medicine is to provide treatments tailored to individual patients. An LLM knowledge graph drug purpose can play a pivotal role in this endeavor by integrating patient data, such as genetic information and clinical history, with drug knowledge. This personalized approach allows for the identification of the most effective and least toxic drug options for each patient, leading to better outcomes.
- Pharmacovigilance and Post-Marketing Surveillance: After a drug has been approved and entered the market, it’s crucial to monitor its performance in the real world. Adverse effects, interactions, and unexpected outcomes may arise that were not observed during clinical trials. An LLM knowledge graph drug purpose can be used to track such data from electronic health records, social media, and patient-reported outcomes, helping to identify and address safety concerns more quickly.
- Biomarker Discovery: Identifying biomarkers for diseases and drug responses is crucial for improving diagnostics and treatments. By analyzing the relationships between genes, proteins, and drugs in an LLM knowledge graph drug purpose, researchers can uncover new biomarkers that could be used to diagnose diseases earlier or predict how patients will respond to treatments.
- Clinical Trial Optimization: Clinical trials are the cornerstone of drug development but are also incredibly costly and time-consuming. An LLM knowledge graph drug purpose can help optimize clinical trials by identifying the most promising candidates based on existing data. It can also aid in selecting the right patient populations, reducing trial duration, and increasing the chances of success.
Challenges and Limitations of LLM Knowledge Graph Drug Purpose
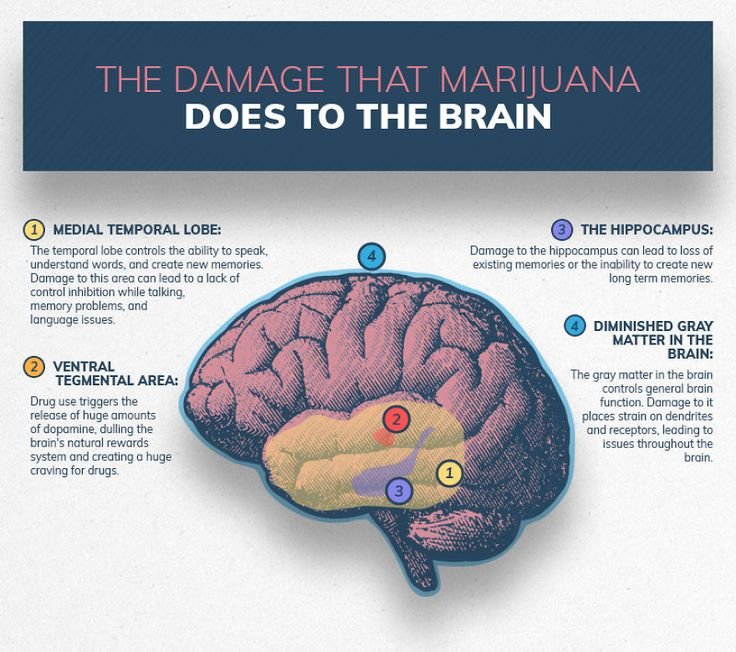
While LLM knowledge graph drug purpose offers immense potential, there are challenges and limitations that need to be addressed:
- Data Quality and Standardization: The effectiveness of a knowledge graph relies on the quality and consistency of the data it processes. Variability in data sources, formats, and terminologies can hinder the accuracy of the insights generated.
- Data Privacy: Given the sensitive nature of healthcare data, ensuring privacy and compliance with regulations such as GDPR and HIPAA is crucial. Researchers must be cautious when using patient data in an LLM knowledge graph drug purpose.
- Model Interpretability: While LLMs are highly effective at generating insights, they can also be considered black boxes. Understanding the reasoning behind the insights and predictions made by the model is important for ensuring that they are reliable and actionable.
- Integration with Existing Systems: For maximum impact, an LLM knowledge graph drug purpose must be integrated with existing pharmaceutical and healthcare systems. Achieving this integration can be complex and time-consuming.
Also read Why They Out Persimmons on Money: A Cultural and Historical Insight
Conclusion
The LLM knowledge graph drug purpose is a powerful tool that is transforming the landscape of drug discovery and development. By combining the capabilities of large language models with knowledge graphs, researchers are able to unlock new insights, optimize existing drug therapies, and streamline the drug discovery process. Despite challenges such as data quality and privacy concerns, the potential benefits of this approach make it an invaluable asset in the search for better treatments and cures for a wide array of diseases. The future of drug development is undoubtedly linked to advancements in LLM knowledge graph drug purpose, offering a glimpse into a more efficient, personalized, and data-driven healthcare system.